A brief introduction
I will document some of my progress in the Deep Learning field, dealing with Biomedical Imaging.
I am currently working on 3D object detection models on Lung Nodules to detect for Cancer.
I have experience in 2D U-Net models for segmentation of Nodules which might be of use for other applications.
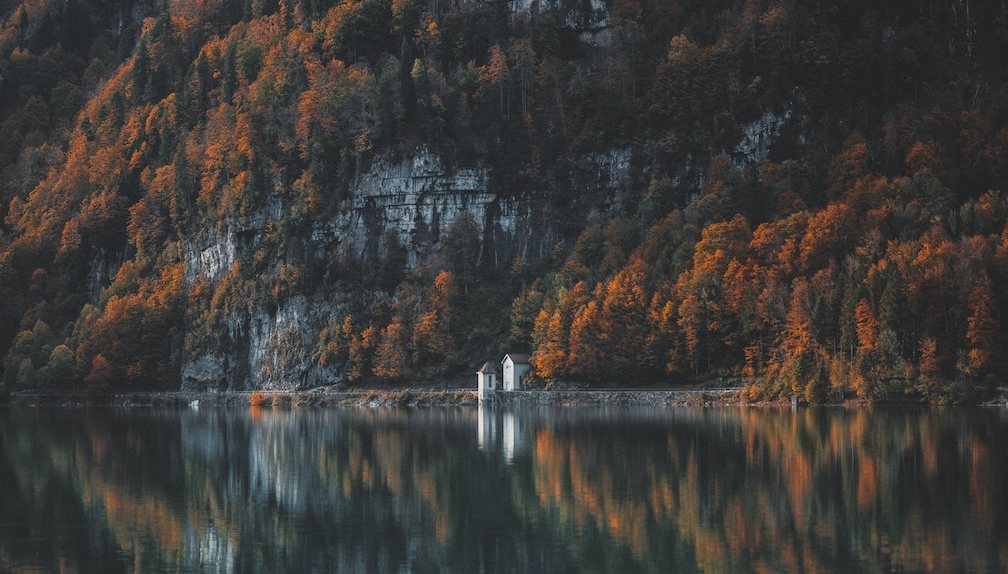
My Story
I'm working on Lung Nodule detection in Computed Tomography (CT) Images in my PhD Research, and wish to be able to contribute more in the biomedical field under Computer Vision. I am currently working on object detection frameworks, which is my main framework being implemented in detecting lung nodules. Previous architecure used was based on segmentation architecture, namely, U-Net model. The base model of U-Net architecture was able to achieve an average accuracy of 70% in detecting lung nodules. Implementation of object detection frameworks have seen an improvement in detection accuracy, which ranges from 85% to 95% accuracy.
It is a personal achievement for myself. I was able to develop a base U-Net model for lung nodule detections with an average accuracy of 70% to 75% from scratch. However, the model was poorly developed and we have decided for a change in direction in approaching the project. As of May 2020, I am in my second year of PhD, and I plan to achieve at least one publication before I enter into my third year of PhD. The current framework revolves around 3D imaging and improvements have been made to existing models which has shown great amount of improvement. Mass simulations are currently being run to collect all possible results on this finding.
Any form of contribution and knowledge sharing, will be greatly appreciated. I am still learning and would be making a lot of mistakes along the way. Some information shared might not be 100% accurate, as it is based on my own understanding on a particular topic. Any form of help to fix these mistakes are welcomed. Enjoy your visit here!